Unleashing the Future: The Critical Role of Training Data for Self-Driving Cars
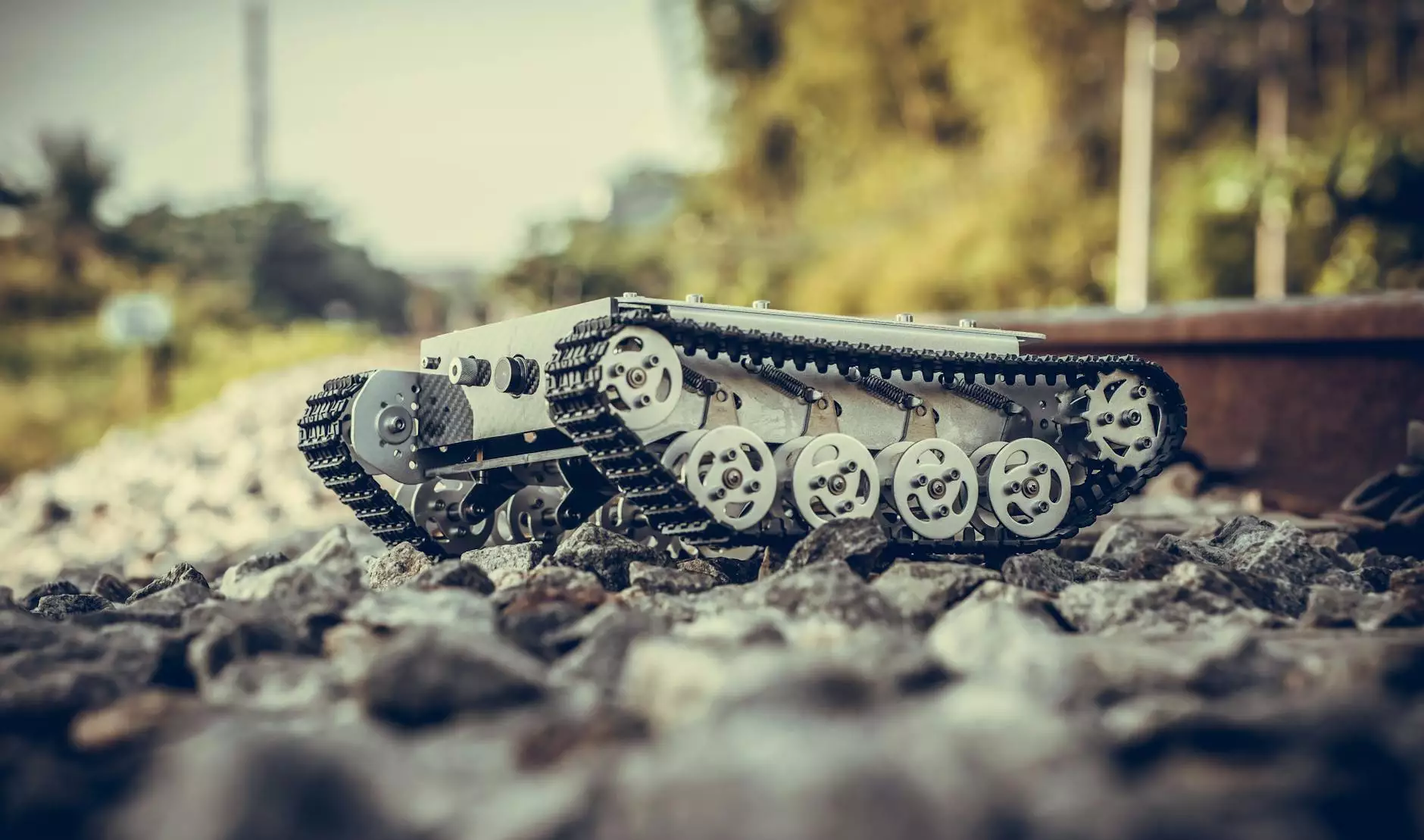
The landscape of transportation is rapidly evolving, driven by advancements in technology and innovative software development. One of the most transformative changes is the emergence of self-driving cars. At the heart of this technological revolution lies the concept of training data for self-driving cars, a fundamental element that enables these vehicles to navigate safely and efficiently in the real world.
Understanding Self-Driving Cars
Self-driving cars, also known as autonomous vehicles (AVs), utilize various technologies to operate without human intervention. Equipped with sensors, cameras, and powerful AI algorithms, these vehicles must process vast amounts of data to make split-second decisions akin to those made by a human driver. The ability to interpret surroundings and respond to dynamic environments is solely dependent on high-quality, comprehensive training data.
The Importance of Training Data
Training data is the backbone of machine learning models used in autonomous systems. It enables the vehicle’s AI to learn from countless scenarios, ensuring safety and reliability. The transition from human-driven to autonomous driving hinges on sophisticated data algorithms that rely on a robust training dataset.
Components of Training Data for Self-Driving Cars
The dataset required for effective training comprises multiple components:
- Image Data: High-resolution images captured from various angles, essential for understanding object recognition.
- Video Data: Continuous footage that provides insights into motion and dynamic interactions between objects on the road.
- Sensor Data: Information from LIDAR, radar, and ultrasonic sensors that help the vehicle perceive its environment.
- GPS and Location Data: Essential for mapping and route planning, providing context for navigation.
- Traffic and Weather Data: Information about conditions and patterns that can affect driving, necessary for adaptive learning.
How Training Data is Collected
The collection of training data is a meticulous process, often involving the following methods:
1. Real-World Driving
Self-driving car companies deploy fleets of vehicles equipped with sensors and cameras to collect real-time data. These cars operate in different environments, capturing diverse scenarios such as urban areas, highways, and rural roads.
2. Simulation Environments
Simulation tools allow developers to create virtual environments where vehicles can experience various driving conditions. These simulations ensure that the algorithms are tested rigorously without putting lives at risk.
3. Public Datasets
Some organizations share anonymized driving datasets that include various traffic scenarios, contributing to collective knowledge in the field.
Challenges in Curating Training Data
Despite the available methods, curating high-quality training data for self-driving cars presents several challenges:
1. Data Diversity
A diverse dataset is crucial to train models effectively across numerous scenarios. This means including data from different geographies, climates, and traffic conditions.
2. Labeling and Annotation
Each piece of data must be accurately labeled to train machine learning models effectively. This process is time-consuming and requires expertise to ensure accuracy.
3. Handling Edge Cases
Edge cases are uncommon or unique driving scenarios that can confuse AI systems. Identifying and adequately representing these in the training data is essential for robust performance.
Role of Software Development in Training Data Utilization
Software development plays an integral role in harnessing training data for self-driving cars. Developers create robust architectures that enable the efficient processing and analysis of large datasets. Some key areas include:
1. Data Pipeline Development
The creation of an efficient data pipeline is paramount. It involves collecting, cleaning, and preprocessing data before it can be fed into machine learning models.
2. Machine Learning Model Training
Developers use various machine learning frameworks to train models on the collected data. Techniques such as supervised learning, unsupervised learning, and reinforcement learning are commonly employed.
3. Continuous Testing and Improvement
To ensure the AI models remain accurate and reliable, ongoing testing is crucial. Software developers implement feedback loops where data from real-world operations is continuously fed back into the training process to improve performance.
Future Directions in Training Data for Self-Driving Cars
As the technology evolves, the methods of gathering and utilizing training data for self-driving cars will continue to innovate:
1. Enhanced Data Annotation Techniques
Automation in labeling processes using machine learning can increase efficiency and reduce the need for extensive human intervention.
2. Collaborations and Partnerships
Collaboration between tech companies, governments, and research institutions will facilitate the sharing of datasets, enhancing diversity and quality.
3. Edge Computing Implementation
Implementing edge computing can reduce latency by processing data closer to where it is generated, enabling more responsive systems for real-time decision-making.
Conclusion: The Future is Autonomous
Looking ahead, the reliance on training data for self-driving cars will only intensify as the industry advances. Through continued investment in software development, data collection methodologies, and innovative technologies, we stand on the precipice of a new era in transportation. The integration of autonomous vehicles promises to enhance safety, reduce traffic congestion, and transform the way we navigate our world.
Keymakr.com is at the forefront of this transformation, committed to providing the technological frameworks and solutions that empower the development of self-driving cars. By understanding the intricate relationship between training data and autonomous driving technology, we can drive forward into a future that redefines mobility.
training data for self driving cars